NeRF Technology: Revolutionizing 3D Rendering with AI-Powered Precision
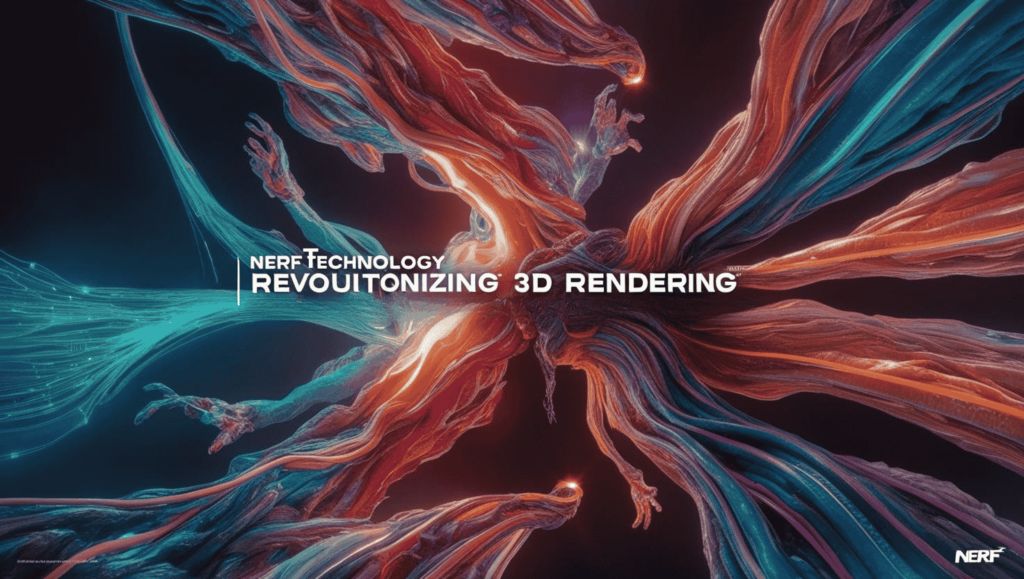
NeRF (Neural Radiance Fields) Technology is an AI-driven method for creating photorealistic 3D models from 2D images. It uses deep learning to understand how light interacts with objects, allowing it to reconstruct highly detailed and realistic 3D scenes.
Unlike traditional 3D modeling, which relies on polygons, NeRF captures depth, reflections, and lighting with precision, making it ideal for gaming, VFX, AR/VR, and medical imaging. This breakthrough technology is transforming how digital environments are created and visualized.
1. What is NeRF Technology?
NeRF is a cutting-edge AI technique for 3D rendering, and video-to-3D AI plays a similar role in transforming footage into 3D models.NeRF (Neural Radiance Fields) is a groundbreaking AI-powered technology that generates photorealistic 3D models from 2D images, transforming the way 3D content is created. Unlike traditional 3D modeling methods that rely on polygons, NeRF uses deep learning algorithms to analyze and synthesize light, textures, and depth, enabling it to produce highly detailed and realistic 3D reconstructions. This makes NeRF ideal for industries like gaming, VFX, augmented reality (AR), and virtual reality (VR), where precision and realism are critical.
By leveraging advanced neural networks, NeRF can capture intricate details such as shadows, reflections, and lighting variations, offering a level of realism that was once difficult to achieve. Its ability to generate accurate 3D models from 2D images opens up new possibilities for designers and developers, providing them with efficient tools to create realistic environments without the need for complex manual modeling. This innovative approach to 3D scene reconstruction is positioning NeRF as a game-changer in the world of 3D rendering.
2. How NeRF Works
NeRF (Neural Radiance Fields) works by training a neural network to predict the interaction of light with objects in a scene, a process that forms the backbone of its photorealistic 3D reconstruction capabilities. Unlike traditional 3D methods, which require complex modeling, NeRF uses deep learning to analyze light, texture, and depth, generating highly accurate and realistic models. By leveraging volumetric rendering, NeRF captures intricate details such as reflections, transparency, and subtle variations in lighting, ensuring that the 3D output mimics real-world scenes with impressive precision.
This ability to reconstruct 3D models from a set of 2D images makes NeRF a powerful tool for industries like gaming, film production, and AR/VR, where realistic environments and immersive visuals are crucial. The volumetric rendering technique allows for the creation of smooth, dynamic 3D models that maintain the quality of the original scene, providing a more seamless and lifelike experience. As a result, NeRF is quickly becoming a preferred solution for high-quality, AI-driven 3D rendering, providing both efficiency and detail in one innovative package.
Example: Imagine scanning a real-world object with a smartphone and getting an ultra-realistic 3D version without needing manual modeling.
3. NeRF vs. Traditional 3D Rendering
NeRF (Neural Radiance Fields) represents a significant departure from traditional 3D rendering methods such as polygon-based models or photogrammetry. Instead of relying on pre-defined meshes, NeRF leverages deep learning to predict how light interacts with objects in a scene, resulting in more natural, photorealistic 3D reconstructions. This AI-driven approach excels in capturing complex lighting effects, reflections, and fine details that traditional methods often miss, especially in dynamic or highly detailed environments. However, NeRF’s heavy reliance on deep learning means it requires significantly more computational power than conventional 3D rendering techniques.
On the other hand, traditional 3D rendering methods are generally faster and have wide compatibility with gaming engines and real-time applications. Polygon-based models or photogrammetry, while effective, often require manual optimization for lighting, textures, and geometry, which can be time-consuming and labor-intensive. While these methods remain a staple in industries like gaming and animation, NeRF’s AI-driven approach is setting a new standard for realism in 3D modeling, especially for projects that demand highly detailed and dynamic visual representations.
4. Applications of NeRF
NeRF technology is making waves across a variety of industries, offering innovative solutions for creating highly realistic 3D environments. In gaming, NeRF is being used to create immersive, lifelike worlds for next-gen games, where photorealism and complex lighting are essential for an engaging user experience. In VFX and animation, NeRF assists in producing AI-powered CGI for movies, enabling studios to create stunning visual effects with more dynamic and realistic lighting. Notably, Pixar and other major animation studios are exploring how NeRF can enhance CGI by delivering more accurate and natural lighting effects, pushing the boundaries of what’s possible in animated storytelling.
In AR/VR, NeRF is revolutionizing 3D world-building by providing immersive, real-time 3D environments that feel authentic and interactive. The technology is also being explored in medical imaging, where it helps generate high-fidelity 3D scans from MRI and X-ray data, offering more detailed and accurate representations for medical professionals. With these applications, NeRF is setting new standards for realism, efficiency, and innovation across multiple sectors, proving to be a game-changer in both creative and technical fields.
5. Advantages of NeRF
NeRF technology offers several significant advantages that set it apart from traditional 3D rendering techniques. One of the most notable benefits is its photorealism. NeRF can recreate incredibly lifelike textures, reflections, and shadows, all without relying on complex shaders, making it ideal for industries that demand high-quality, realistic visualizations. This ability to simulate real-world lighting and depth makes NeRF a powerful tool for generating visually rich environments, especially in areas like gaming, VFX, and AR/VR.
Another key advantage of NeRF is its automation. The AI-powered scene generation dramatically reduces the manual labor typically required in 3D modeling. Instead of manually creating and optimizing 3D meshes, NeRF learns from 2D images to generate detailed 3D reconstructions, which increases efficiency and accelerates the design process. Additionally, NeRF excels in depth and light simulation, capturing lighting variations more accurately than traditional 3D rendering methods. This results in more realistic environments, enhancing the overall quality of the final output.
6. Limitations of NeRF
Despite its impressive capabilities, NeRF technology comes with a few notable limitations. First, it is computationally expensive. NeRF requires powerful GPUs and significant computational resources, which can make it less accessible for users with limited hardware. The heavy reliance on deep learning and AI models demands a lot of processing power, making it a costly option for smaller studios or individuals working with tight budgets.
Another limitation of NeRF is its slow processing times. Unlike real-time rendering engines, which can generate scenes almost instantly, NeRF takes longer to process and generate high-quality 3D models. This can be a drawback for industries that require fast turnarounds, like live broadcasting or fast-paced video game development. Lastly, NeRF also has limited editing flexibility. Once a scene is generated, modifying it can be challenging, as it doesn’t offer the same level of flexibility as traditional polygon-based 3D models. Making changes or adjustments to the scene often requires re-training the model, which can be time-consuming and resource-intensive.
7. The Role of AI in NeRF
The role of AI in NeRF (Neural Radiance Fields) is central to its ability to generate photorealistic 3D models. NeRF leverages deep learning and neural networks to predict how light interacts with objects in a scene. By analyzing large image datasets, AI models can learn the complex relationships between light, textures, and depth, allowing NeRF to reconstruct a fully immersive 3D view. The neural network processes volumetric data to generate highly detailed visual outputs, making it ideal for applications that require accuracy and realism, such as virtual environments or digital twins.
An example of NeRF’s AI capabilities can be seen in Google Research, where AI-powered NeRF is being used to improve street-level mapping in Google Earth. By leveraging AI, Google is able to create more accurate, realistic representations of real-world environments, making virtual navigation and exploration more lifelike. This showcases how AI-driven NeRF technology is transforming industries by providing more detailed and efficient 3D reconstructions, revolutionizing everything from mapping to gaming and medical imaging.
8. NeRF Training Process
The NeRF training process is a critical step in generating photorealistic 3D models. It begins with the input of multiple images of a single scene from various angles. These images serve as the dataset that the AI model uses to understand how light interacts with the scene and how depth is perceived across different perspectives. By analyzing this data, NeRF’s neural network learns to predict color and density for every pixel, which is essential for creating a seamless 3D reconstruction.
Once the AI model has been trained to understand the lighting and depth information, it proceeds to render the output — a fully reconstructed 3D model that accurately replicates the original scene in a photorealistic manner. This process allows NeRF to generate detailed models that can be used in applications like gaming, AR/VR, and virtual production. While the training can be computationally intensive, the end result is a highly immersive and realistic 3D environment that traditional methods struggle to replicate.
9. Volumetric Rendering in NeRF
Volumetric rendering is a core feature of NeRF, and it plays a significant role in how the technology creates photorealistic 3D models. Unlike traditional polygon-based 3D models, which are built from predefined meshes and vertices, NeRF simulates how light travels through 3D space to capture more natural lighting effects. This process allows NeRF to generate soft shadows, accurate depth perception, and realistic reflections, resulting in visuals that are closer to how we perceive the world in real life.
An excellent real-world example of volumetric rendering is how fog effects are created in movies. In these scenes, light is simulated as it scatters through fog, creating a soft, atmospheric look. NeRF uses a similar approach, simulating light scattering and interactions with various materials in a 3D scene. This leads to richer, more dynamic visuals that traditional polygon-based models can’t achieve, making NeRF ideal for applications where visual realism is key, such as in gaming, VFX, and AR/VR.
10. NeRF and Computer Vision
NeRF and computer vision are becoming increasingly intertwined, with NeRF enhancing how machines interpret and understand the world around them. By generating photorealistic 3D environments, NeRF significantly improves object detection, scene understanding, and 3D environment mapping. The AI-powered models enable machines to “see” depth, texture, and light interactions in a more sophisticated way than traditional computer vision technologies. This makes it easier for AI systems to process and analyze complex visual data, leading to more accurate decision-making.
An excellent real-world example of NeRF’s impact on computer vision is Tesla’s exploration of NeRF-based AI to improve their self-driving car vision systems. By leveraging NeRF technology, Tesla is enhancing its cars’ ability to recognize and interpret 3D environments in real-time, which is crucial for navigating safely in complex driving conditions. This application showcases how NeRF is not only advancing industries like autonomous driving but also playing a key role in the future of intelligent
11. NeRF in Cloud Computing
NeRF in cloud computing is a game-changer for industries that rely on 3D rendering but lack access to high-end hardware. Cloud-based NeRF solutions allow users to access AI-driven 3D rendering capabilities remotely, eliminating the need for powerful on-site machines. This opens up opportunities for game developers, architects, and VFX artists to generate complex NeRF models and render detailed 3D environments without investing in expensive computing infrastructure.
By utilizing cloud services, professionals can run resource-intensive NeRF processes on scalable servers, which makes real-time rendering and 3D scene creation more accessible. This not only reduces costs but also accelerates workflows, allowing creative teams to focus on their projects rather than worrying about hardware limitations. For instance, architects can remotely generate realistic 3D models of buildings and landscapes, while VFX artists can create immersive environments for films and games, all from cloud-powered NeRF tools.
12. Real-Time NeRF Rendering
Real-Time NeRF Solutions are transforming the way 3D environments are generated, making instant, photorealistic rendering possible. Companies like NVIDIA are pushing the boundaries with GPU acceleration and optimized AI models to enable real-time NeRF rendering for AR, VR, and gaming. This means that instead of waiting minutes or hours for 3D scenes to be processed, users can experience dynamic, AI-powered environments instantly.
These advancements will have a major impact on industries like metaverse development, where immersive, interactive worlds require seamless real-time 3D generation. For example, game developers will be able to create lifelike game worlds on the fly, while AR/VR applications will deliver instant scene reconstruction for virtual tourism, training simulations, and interactive storytelling. As real-time NeRF technology matures, it will become a core component of next-gen digital experiences.
Final Thoughts
NeRF technology is revolutionizing 3D rendering, AI-driven content creation, and immersive virtual experiences. By leveraging deep learning, NeRF can generate highly photorealistic 3D models from simple 2D images, eliminating the need for complex manual modeling. This innovation is already making waves in gaming, film production, AR/VR, and the metaverse, offering unprecedented realism and automation in digital content creation.
Despite challenges like high computational costs and slow processing times, ongoing advancements in GPU acceleration and cloud-based rendering are making NeRF more accessible. As the technology evolves, it is set to transform industries reliant on 3D visuals, providing a faster, more accurate, and AI-powered approach to building lifelike virtual worlds.